Adjacent clusters in Google shopping – designing your strategy for smart bidding
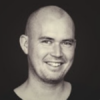
Tobias Hink
Data Solutions Engineer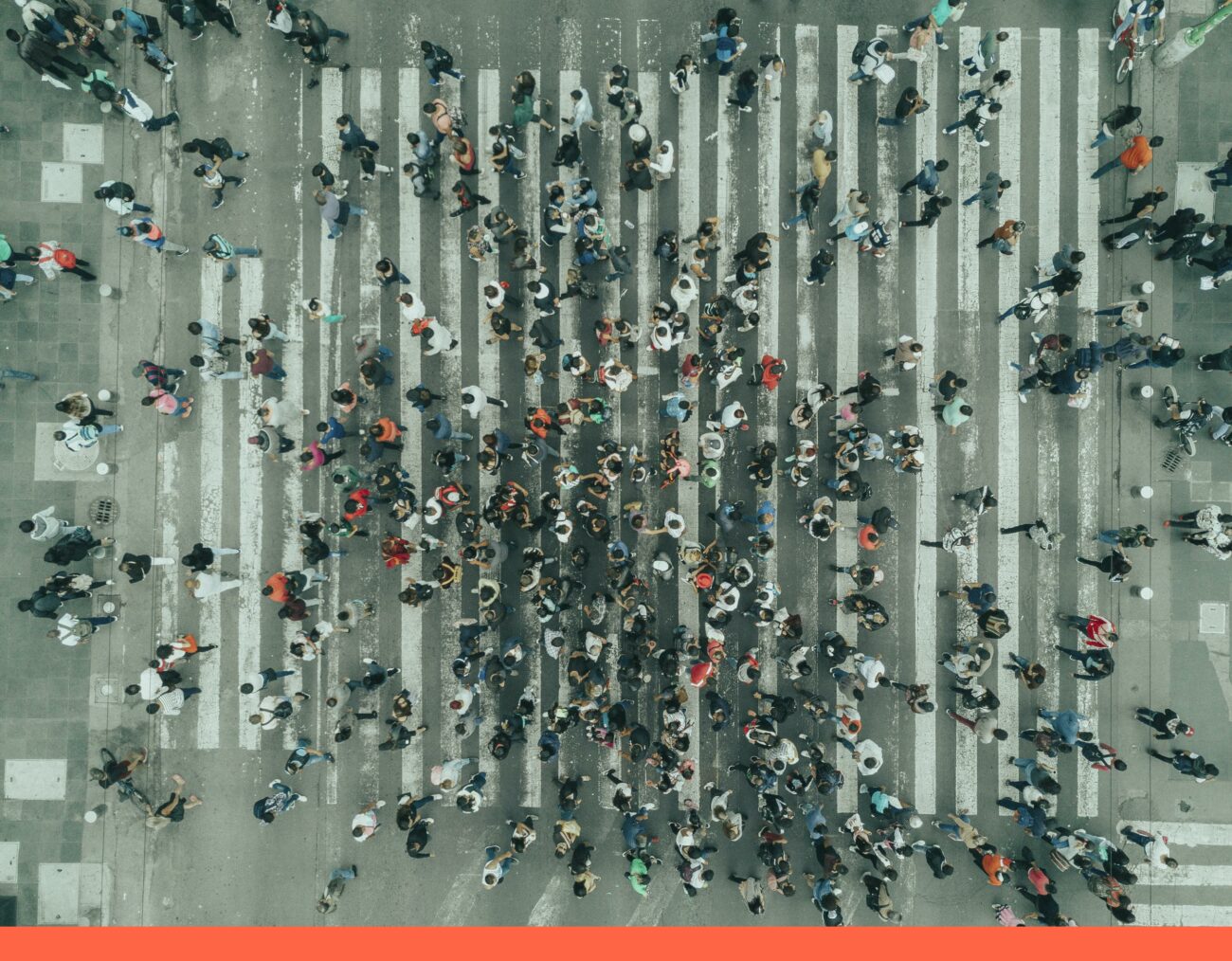
So you are running shopping campaigns with smart bidding, and it is not performing as you might want it to… Many retailers rely on Google’s Smart Bidding to handle bidding across large product inventories on Google Shopping – but just how smart is Smart Bidding actually? In this article, we explain some of the mechanisms behind Smart Bidding for Google shopping and suggest a practical approach to maximizing efficiency for your inventory.
We recently wrote about how investments in search among retailers have shifted from regular search ads to Google shopping over time, and how to achieve operational excellence at scale. You can read that article here.
As the overall investment from advertisers in Google Shopping increases, the auction intensifies, and the need for efficient bid and inventory management to stay competitive on Google Shopping becomes absolutely crucial.
One possible solution for bid management, is Google’s own Smart Bidding that promises to “save time and improve your return on investment.” Google Smart Bidding is an automated bid strategy, that automatically place auctions based bids based on the target of a portfolio, campaign or ad group on your behalf. Smart Bidding is often the first choice for managing Google Shopping campaigns due to the wide range of contextual signals that Google can access together with the Advanced machine learning capabilities it holds.
If you are new to Smart Bidding I suggest reading Google’s own guide to smart bidding here.
How smart is Google’s Smart Bidding?
Smart bidding for regular search ads and Smart bidding for Google Shopping are two very different things
Smart bidding for shopping relies on a feed provided by the business owner with valuable information such as price, GTIN etc. so that Google can better predict the potential value of the next click, giving it an advantage over regular search ads, that mostly rely on historical query data and performance.
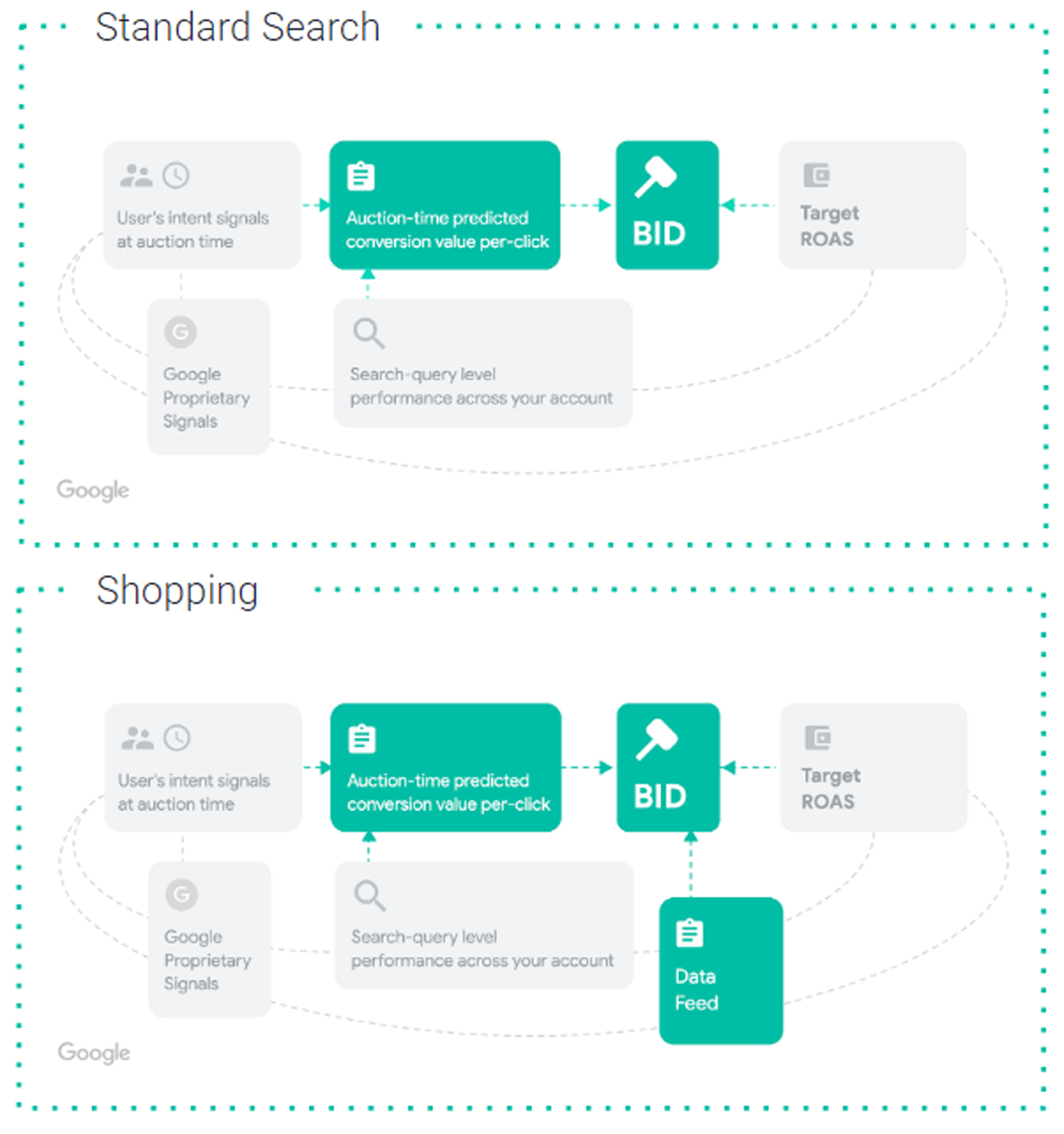
Shopping is better at identifying value per click or expected average order value due to the feed based information from the merchant centre.
Smart Bidding will in most cases deliver the desired set target ROAS (Return on Advertising Spend) over time, and It outperforms most manual and 3rd party bidding solutions when it comes to COS (Cost of Sales)/ROAS optimization for Google Shopping, but how does it actually treat your inventory?
The simple version of how the CPC is calculated based on a set ROAS target is:
Or even simpler:
Predicted value per click is then decided by signals of user intent, Google proprietary signals (previous visited websites, detailed demographic data, purchase history) and Search Query performance across the account. Due to the nature of how the auction works, leverage is added because the actual cost of the click is not necessarily the same as the bid.
For Google Shopping, the ROAS formula could look like the example below where feed data could be a factor around +-1, based on level of competition, prices etc., but this is only a theory.
Even though we know that feed data most certainly plays a role in predicting value per click, we don’t know how Google actually utilize it in their algorithms.
To summarize: Smart Bidding for Google Shopping is better at predicting the value of the next click than for regular search, however the predicted value for the next click relies on many factors other than the actual product advertised.
Below is a visualization of how the distribution of a product set revolved around the campaign target, when setting a target ROAS of 500% for a selected product inventory within one target setting.
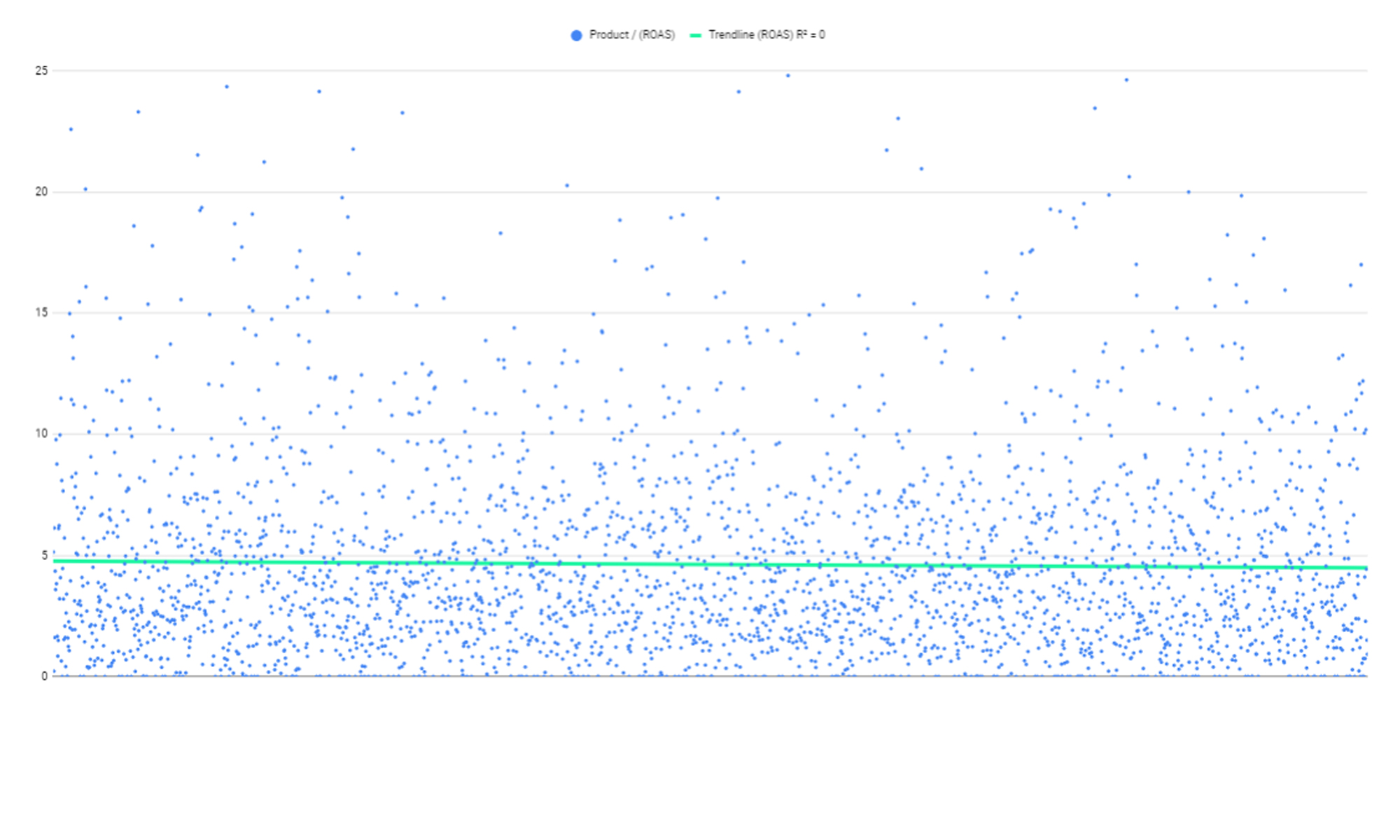
Each blue dot represents a unique product and its ROAS over 1 year, with a randomized horizontal placement. The green line represents the average overall ROAS among all products in the campaign.
The important lesson here is that Smart Bidding is not very good at granular bidding when looking at individual products only – at least not if you bulk everything together: It doesn’t tell us any true story about the products – other than Google cannot deliver a good average individual product level ROAS .
The graph above solely represents performance at the individual product level. As previously mentioned, Google uses a ton of signals for Smart Bidding, including: search queries, audiences, devices, location etc.
When you bulk all signals together, you might see a more granular model with fewer outliers, than the one we see for the products above.
However, if your goal is to achieve more granular bidding across your entire product portfolio in order to obtain an average ROAS for each product, or evenly distribute impressions (thus achieving to maximizing sales across all products), an adjacent cluster approach might be the answer.
Adjacent clusters in Google Shopping
“Adjacent means close to or near something. You may consider the people up and down your street to be neighbors, but your next-door neighbor is the person who lives in the house or apartment adjacent to yours.”
To understand the logic behind adjacent clusters in a Google Shopping setup, we need a framework to understand the possibilities within shopping campaign management. There are many ways to utilize the campaign settings and priority levels. At Precis we believe that the most intuitive framework is to use priority levels to segment search queries and utilizing the campaign inventory filter for product segmentation.
Combining these two into a strategy can be tricky due to the necessity of correct product markup data, and mutual search query exclusions that can occur when handling multiple campaigns at multiple levels.
To get a simple idea of the framework, it can be visualized it in a classic X-Y coordinate system that could look something like this:
In principle, the product segmentation can be anything that defines your inventory, e.g.: verticals, margins, CLV (customer lifetime value), popularity (internal), price or expected avg. order value.
The same applies to search query segmentation. As long as it stays within Google’s 3 priority levels (high, medium, low), you can really let your imagination fly. Examples of search query segmentation are similar to those of the product segmentation. The segmentation should align with the business strategy and current priorities, whether that is to maximize revenue or profit, secure even sales across your entire inventory, or bolster the sales of particular, seasonal products.
You can go even more granular by utilizing overrides at the ad group level in combination with product subdivisions – however, keep in mind that each cluster should have sufficient data to unlock the power of Smart Bidding (Google suggests that a Target ROAS strategy requires more than 50 conversions over the span of est. a month or longer to be able to evaluate results correctly).
The purpose of doing this kind of segmentation is to set better targets and maximize sales, while maintaining a desired ROAS target within all products- and search query clusters.
People don’t (always) buy the products advertised…
A study posted on Search Engine Land from 2016 shows that only 34% of converters bought the product advertised in the ad they clicked on from Google shopping. E.g. When segmenting your products based on margins, a single product on google shopping may have high margins (thus high CPC), and driving a lot of conversion, but customers end up buying something completely different, thus making margins (yield) redundant as a segmentation criteria for managing yield.
An internal study at Precis indicates that this can vary greatly between businesses, and especially between single and multi-brand websites. For a major single brand website we found a correlation where up to 71.6% of converters bought the product they actually clicked on in Google’s results.
Nonetheless, segmentation for setting correct targets is more important than ever, but instead of optimizing bids on products 1-1, one should evaluate every product as a data point that contains many signals and variables that reflect the performance of the business, and cluster them accordingly to make Smart Bidding work for you.
Question what we know today and embrace change…
Managing a large inventory with variance in performance, stock levels, margins etc. requires great target settings. Letting Smart Bidding decide what is best for your business by bundling everything together with a single target is risky.
The adjacent cluster setup works for all types of businesses, but the actual campaign structure depends on a range of factors: Is the business selling a single or multiple brand(s)? How is the competitive landscape? Is the goal to drive revenue, profit or CLV?
To achieve the best possible setup for your business, proper testing is needed. The correct hypotheses and theories need to be in place prior to any experiments. The best place to start is by looking at historical data to find answers to one of more of the following exemplary questions:
Where are the biggest inefficiencies pertaining to cost?
- Is there room for increasing impression share, click share and abs. Top impression share?
- What is the Impression share for certain key products or search queries?
- What verticals or queries are losing/gaining traction year on year?
Next step is to plan the test. Google does not support any type of campaign experiments for Shopping, but it is possible to achieve something close to a true A/B test with 3rd party tools. At Precis we have managed to create a solution that simulates A/B testing based on your feed. The tool randomizes between products based on different performance metrics and can test any changes on both the control group and the test group on intervals based on a selected frequency. It’s feed based and applied through supplemental feeds in the Google Merchant Center, and can be utilized to Perform feed testing (e.g. titles etc.) as well as campaign testing (product segmentation). If you do not have access to our tool or similar tools, it could be a period-on-period testing, or simply separating a few selected clusters from the current campaign setup. Finally, evaluate the results, gather learnings and redo the process.
Smart bidding will usually do what you ask it to, but consider if you are asking for the right thing? No algorithm is better than the data you feed it.
Setting the right targets is a tough task, but the adjacent cluster setup is a great first step to provide better insights and target settings for selected clusters.
Conclusion
We would love to learn from your thoughts, questions and experiences, so feel free to reach out to start a further discussion at hello@precisdigital.com.