Inspiration from Silicon Valley
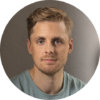
Christoffer Lötebo
Group CEO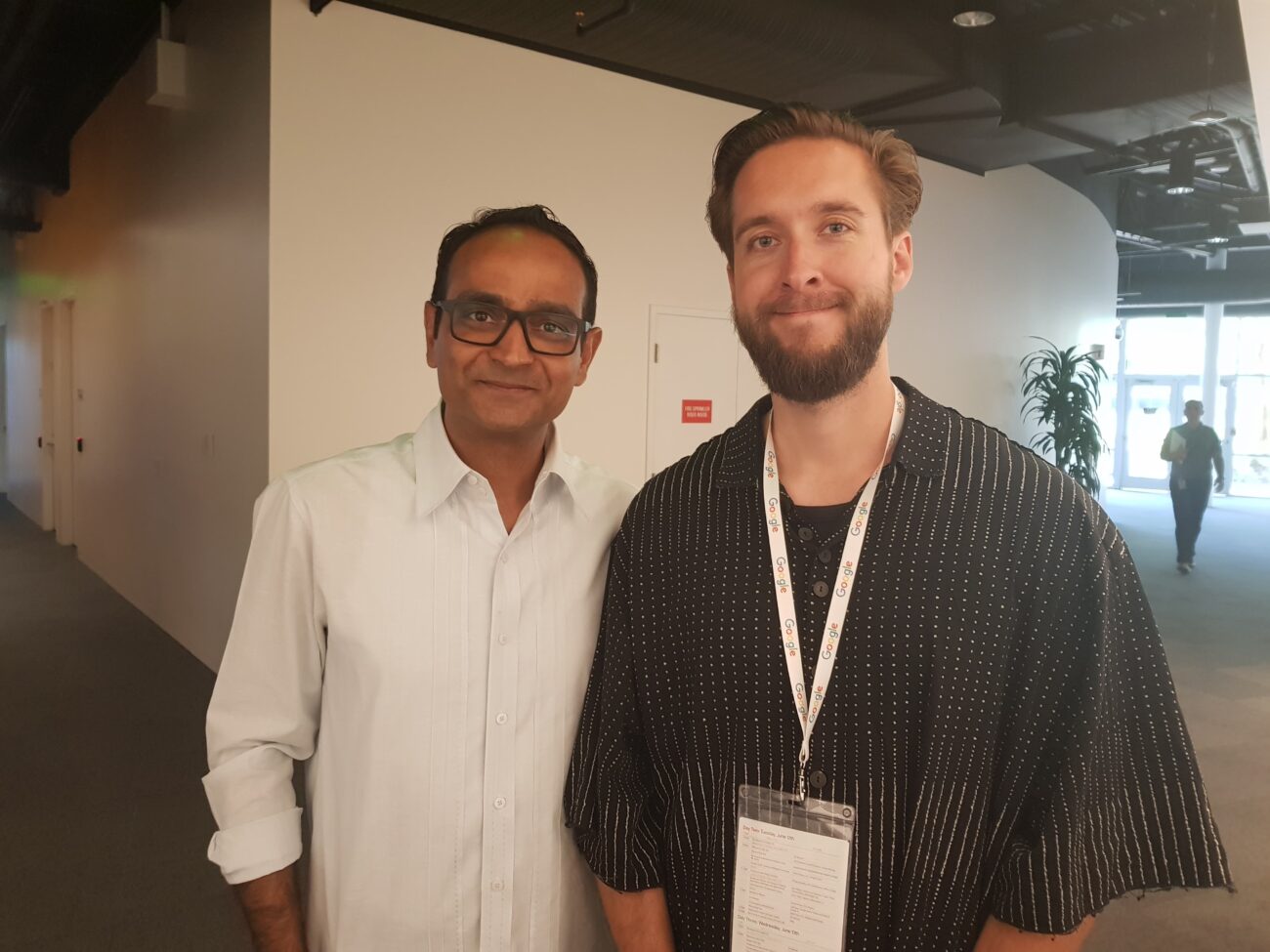
This week we represented Precis at the yearly Google executive management tour, together with participators from nine European countries.
The purpose of the conference is to look beyond the buzz and provide a behind the scenes peek into how the tech industry is progressing and bring some perspectives on if, why, and how they will affect us, society, and businesses as we know them. This years’ conference was held at the Googleplex in Palo Alto.
The conference was packed with exciting speakers and topics. To name a few of them, we got the opportunity to listen in on Pascal Finette, Hod Lipson, Ruth Porat, Astro Teller, and many more…
The sessions covered technological advancements in exciting areas such as blockchain, robotics, longevity, cloud, and machine learning. In this blog post, we will limit ourselves to the latter.
Machine learning is already everywhere, and it’s accelerating! The development over the past years has been tremendous. As an example, back in 2012, image recognition algorithms had error rates of 15%-20% when performing such as “simple” task as identifying a cat. Most you might know about the “Chihuahua or Muffin” case. That’s no longer an issue. Already in 2017 error rates for image recognition was down to 3%. That’s, in fact, better than image recognition performed by humans. And let’s skip the whole AlphaGO thing for now. According to Hod Lipson, this development has been unlocked by mainly four elements:
- Moore’s law: Technology advancements has, and continues to, follow Moore’s law. This results in big decreases in cost of for instance computing power, due to exponential improvements.
- Math: Mathematical breakthroughs have enabled machine learning experts to add more neural nets to their learning algorithms, reaching [very] deep learning.
- Data: Data is fuel to machine learning and let’s just say we’re getting more of it.
- Cloud: Developments in cloud technology allows machine learning systems to teach each other and to scale. Waymo’s self-driving cars is a good example of this. Each car teaches all other cars based on its experiences, enables through a central ”mind” in the cloud.
There are many potential applications of machine learning to existing problems. Apart from applications in self-driving cars, healthcare, and agriculture, which is arguably more important, it also unlocks a great potential when it comes to marketing. It can be applied to problems such as bid management, ad testing or even creative, but maybe most interesting is the potential in audience analytics, as in analysis and action based on consumer data. Machine learning can be used to segment consumers in real-time and reach them more efficiently in media channels as well as serve personalised content. We believe that the impact from this will be huge, and apart from improving users’ experience through increased relevancy, it is also likely to lead to a reduction in marketing waste. All in all, machine learning can be applied to both increase revenue and decrease cost at the same time – well hello ROI of the 21st century!
An example of this is the personalisation of a websites’ content. A website that is personalising its content based on the previous behaviour will be more efficient in tailoring the experience for each user. Since that represents an improvement for both the consumer and the website owner, we’re talking full out Pareto efficiency! Yep, personalising the content based on the previous behaviour sounds like an obvious one, but the fact is that a majority of websites still have not unlocked this potential. Avinash explained it well by showing a website that presented hundreds of different products in many categories, even though he had visited the website many times and on purpose expressed clear interest for a certain product category via behaviour. He compared this experience with the offline retail world to prove an important point:
“It’s like walking up to an employee at a grocery store and ask for milk, and getting the answer: – Here is the key to our warehouse – go f*ck yourself.”
The same logic can be applied for investments in marketing channels. With proper use of existing data through machine learning, there’s no longer an excuse to push the exact same message to everyone, even if it’s an “upper funnel” activity.
We are well aware that reaching full personalisation based on machine learning is challenging as well as investment heavy, but it is clear that having it as a cornerstone in the long-term marketing strategy is important. As with all new features and tactics of marketing, they have the potential to deliver a competitive advantage for the ones that adopt early. Arbitrage is profit. On the flip side, they can come with bad news for the ones that adopt too late.
With all this inspiration with us, we are even more excited to keep on applying to exist, as well as developing new, methods to utilise machine learning to transform marketing for the better.