Search 2020 – Algorithms and the meaning of value (Part 2)
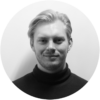
Andreas Nguyen Arentoft
Marketing Science Director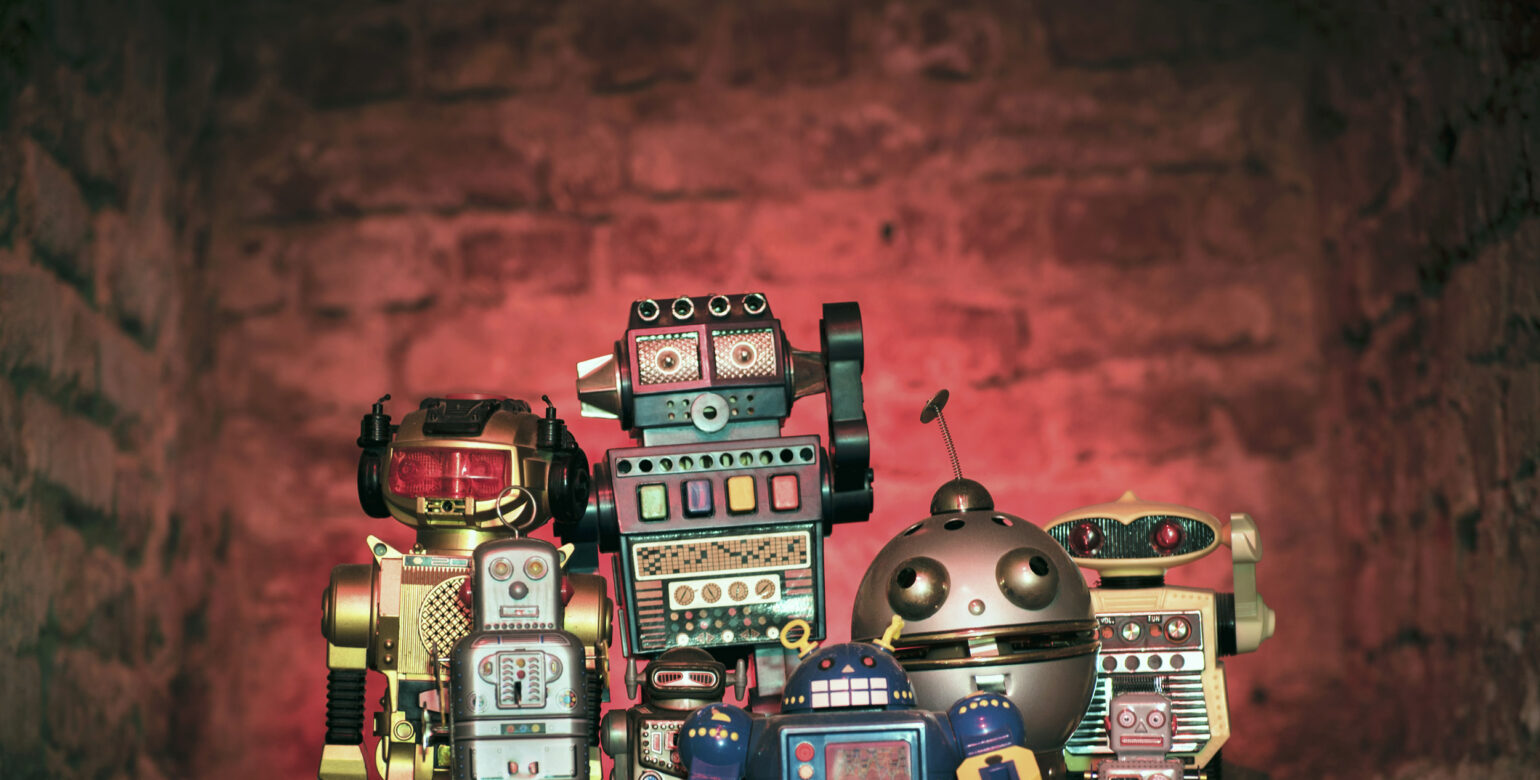
In the first post in our Search 2020 series we discussed the transformation Search marketing has undergone over the last couple of years and introduced the critical skill areas marketers must master to succeed in today’s landscape. It is now time to take a deeper look at one of those areas: how to align your business strategy with the algorithm. To achieve this, marketers will need to improve their understanding of strategic planning and at the same time acquire technical skills within data modeling and data integration. In the end, businesses will find it much easier to find relevant customers and focus on maximising long-term value instead of short-term sales.
Today, most Search campaigns are partly or fully run using algorithms and machine learning. This includes everything from bidding, delivery, and even copywriting. Given the nature of Search marketing this is a natural evolution. As predicted in 1949 by Norbert Wiener, potentially the first person to theorize about AI:
If we can do anything in a clear and intelligible way, we can do it by machine.
But even the smartest machine cannot (yet) guess the objective you want to achieve, nor understand fully if it is successful. A machine learning algorithm is like a dog. You ask it to do something, it tries to follow your command, and you give it a treat if the action matched what you wanted it to do (read more here). It is the marketer’s job to decide when to give the “treat”, in other words, send the right signals to the algorithm when it brings about the desired outcome.
In this post we will address the considerations Search marketers should make when designing a bidding strategy that is aligned with the overall business goal. The end result will be to teach the algorithm what it needs to optimize towards. However, before we can start rewarding “good” behaviour, we need to know ourselves what “good” actually means.
Who is valuable to you?
Defining your strategic priorities
Even the most technically advanced solution is only as good as the strategy behind it, so marketers need to focus on what really matters. Some businesses might employ short term thinking, but the ultimate marketing strategy is one that prioritizes long-term value over short-term growth.
A sale is not just a sale. The first time a customer experiences a product is the most important and unique opportunity to forge a long-term relationship. Marketers should optimize towards increasing the lifetime value of their customers rather than sales this quarter. As Nicolas Darveau-Garneau, Chief Search Evangelist at Google, puts it:
Short-term thinking yields short-term results.
Search marketers must also account for the fact that algorithms still struggle to optimise towards more than one outcome. For example, balancing efficiency (ROI) with growth (sales volume). Whereas human analysts naturally balance objectives, the algorithm needs clear directives. It is therefore important for marketers to establish a hierarchy of objectives. This becomes increasingly important as marketers adopt Google’s Smart Bidding where only one objective can be selected. (For more details the different Smart Bidding strategies, we recommend this article).
Once you have outlined the exact purpose of your Search marketing strategy and know what you want to optimize towards, the next step is to understand who your marketing activity should target. Previously, marketers have focused on identifying user intent through what is searched for, i.e. which keywords a user triggers. However, since Google Smart Bidding has enabled query-level bidding, marketers can direct their energy to defining who exactly their customers should be.
Identifying relevant customers
Identifying who drives value for your business is an art that marketers must master. The best way to create a long-term strategy is by investing in relevant customers.
If a business does not focus on finding relevant customers and simply tries to maximize their short-term sales, they will find themselves having paid a lot for customers who are not likely to use their service again. A relevant customer is much more likely to be a happy customer, and happy customers return. We refer to this as customer-centric marketing.
To execute a customer-centric marketing campaign, marketers need to identify:
1. Who are their most valuable customers?
2. What are the commonalities among them?
Exactly what those commonalities are will be almost unique to every company, and marketers must again utilize their understanding of the business to guide them in their investigation. There is really no limit to the kind of factors that could potentially determine the value of a customer, so marketers need to exercise a healthy amount of focus to avoid analysis-paralysis.
There are generally speaking three types of data that marketers need to look at:
- Lifetime value: The accumulated value (sales) of a customer since the first purchase.
- Attributes: Static features, such as demographics, device and location data.
- Behaviour: Users behaviour before and after conversions.
The measurement of the lifetime value of a customer and their behaviour requires marketers to collect data over multiple user interactions and this requires consent. Companies might have to work with an incomplete picture since only a subset of users are likely to opt-in to having their data used for marketing purposes. Marketers must therefore build their data models by extrapolating the insights from the high quality data to the users in their “blindspot”. For a deeper discussion about user data and consent, read our blog post: What is a user anyway?
For marketers who can identify their most relevant customers, the next step is to define the criteria for a successful customer acquisition, specifically, setting the proper targets and budgets for different customer groups.
Designing the criteria for success
As mentioned, the algorithm only understands what success looks like if marketers tell it. One of the ways marketers can achieve this is by segmenting users or interaction types into “Value Buckets”. A Value Bucket is a grouping of users that has shared traits and expected lifetime value. By doing so, you will be able to tell the algorithm both who is a high-value customer and also who is likely to contribute very little to the business. This will enable us to set more specific targets for each segment that in turn will improve the efficiency of our investments.
However, marketing does not happen in a vacuum. Since Search ads are predominantly shown to users with an existing intent to buy, the channel can be over-credited when compared to other media. Targets and budget allocation must account for this effect in order to harmonise investments and maximise cross-channel performance. As such, you should use insights gleaned from path-based attribution, incrementality studies, and media mix models to send more realistic signals to the algorithm. For a more lengthy discussion about attribution and incrementality, check out our previous post on the topic.
With the strategy, user insights, and targets/budgets mapped out, it is time to teach the algorithm what it should optimize towards.
Teaching the algorithm
There are two ways to tell the algorithm who your most relevant customers are:
1. Value Bucket Segmentation: Segment your customer into different Value Buckets by using campaign settings, and set different targets, bid and/or budget based on their estimated lifetime value.
2. Custom Conversion Uploads: Use offline conversion tracking to adjust the conversion value of conversion based on estimated lifetime value.
Value bucket segmentation is an elegant solution due to its simplicity and easy implementation. It is therefore likely that this will be the go-to method for most marketers. If you use different targets and/or budget for campaigns with different targeting (keywords, location, device etc.), you are already doing value bucket segmentation. However, this only scratches the surface of what is feasible and marketers with a deep understanding of how to use keyword groupings, target setting and bid strategies can build account structures designed to maximize long-term value.
This method alone has however quite a few limitations since 1) it only allows user segmentation using identifiers available at the time of the query and 2) segmentation is limited to platform settings. This ignores the usage of user behaviour data as signals to the algorithm, as well as being difficult to modify on an ongoing basis.
Conversely, Custom Conversion Uploads is great for marketers seeking a more dynamic solution. By using offline conversion tracking to adjust conversion values rather than changing account settings, marketers gain much more freedom in terms of the signals they can give the algorithm. Marketers can incorporate user behaviour data into the conversion value estimation even if it happens days, weeks, or even months after the user clicked on an ad.
Custom Conversion Uploads can also account for far more complex data modelling, as well as combining CRM data dynamically. This method works with non-linear prediction models, such as Neural Networks and Random Forest, making it extremely flexible and potentially very precise. Having such a powerful tool in the arsenal will make marketers capable of truly teaching the algorithm who their most relevant customers are.
_
Final thoughts
Teaching the algorithm how to optimize towards relevant customers is not easy and marketers are right to be daunted. Nevertheless, in order to evolve with the industry, marketers need to start working more strategically with the signals they give the algorithm and acquire the skills required make it happen. Rome was not built in a day, but remember:
If you can’t fly then run, if you can’t run then walk, if you can’t walk then crawl, but whatever you do you have to keep moving forward.
In the next post in our Search 2020 series, we will focus on the Search marketer’s role in the organization and how there is a need for them to adopt a broader set of responsibilities. Read it online 19/02/2020.