How to collect, store and activate data in marketing
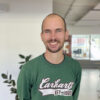
Anton Tikjøb Hagedorn
Data Strategy Lead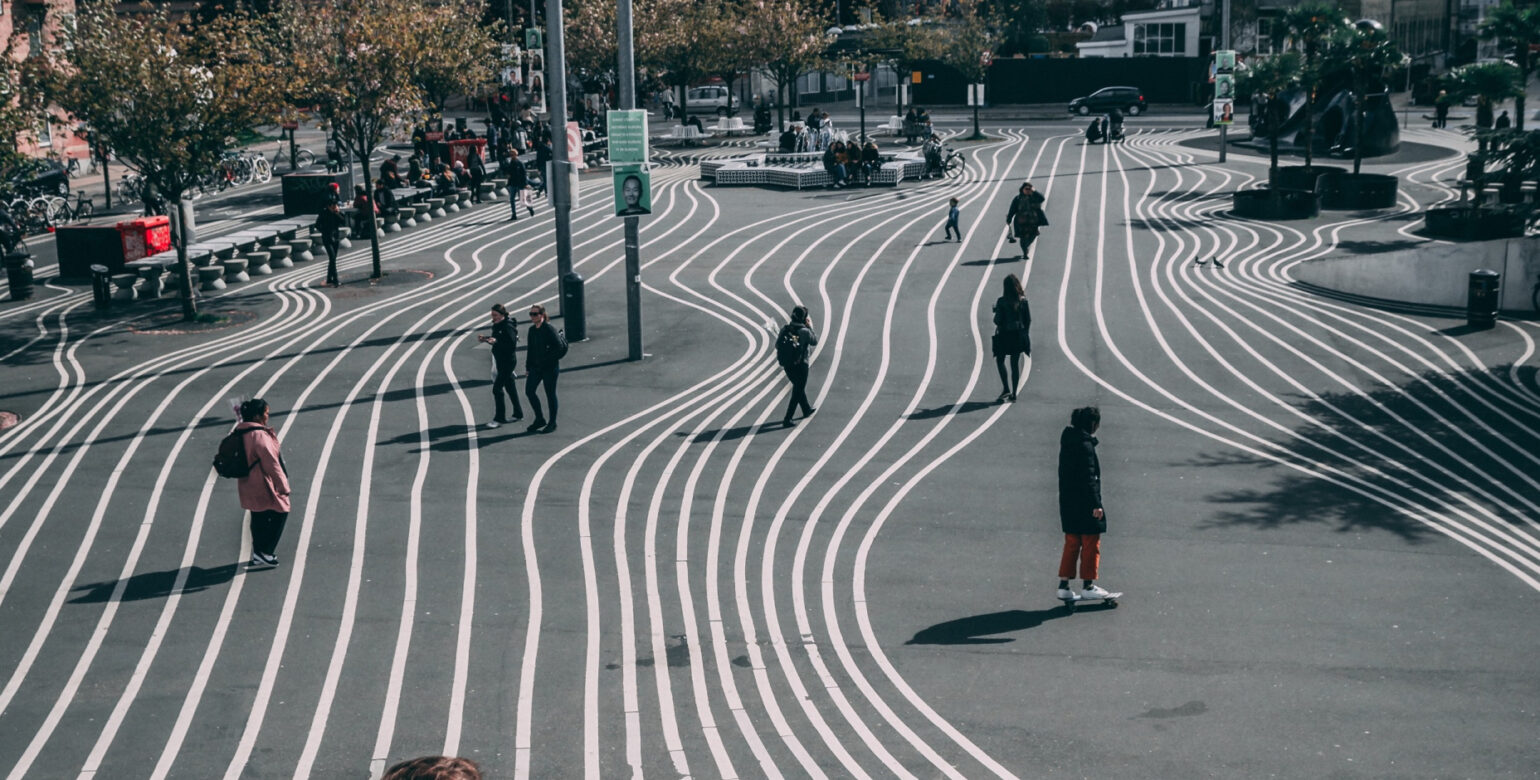
Here’s a comprehensive overview of the intricate world of data collection, storage, and activation in marketing. We understand that the topic may seem complex at first, but fear not! We’ll break it down into bite-sized pieces, making it easy for you to grasp.
The key to successfully navigating this vast landscape lies in aligning all the individual pieces to a shared goal or what we call a “North Star” KPI. By doing so, you’ll be able to unlock the true power of marketing data and leverage it to make informed decisions and drive success in your marketing endeavours.
So, whether you’re a seasoned marketer or just starting, this guide is here to equip you with the knowledge and strategies you need to collect, store, and activate data effectively to guide you towards your North Star.
1. How to collect data
1.1. What is data collection?
Data collection is the systematic process of gathering relevant and valuable information from diverse sources. It involves collecting details, facts, and data points from multiple channels and platforms. This process is crucial in marketing as it enables businesses to understand their target audience, their behaviours, preferences, and other factors that can impact important business decisions.
By thoroughly collecting and analysing this information, organisations can gain valuable insights and make informed choices to drive their marketing strategies towards success.
1.2. Why is data collection important in marketing?
In today’s competitive market, understanding consumer behaviour is crucial for marketing success. Data collection plays a vital role in this process by providing valuable insights for informed decision-making. By systematically collecting data, businesses can uncover patterns, trends, and preferences among their target audience.
One key benefit of data collection is the ability to tailor offerings and personalise experiences for customers.
- Analysing collected data helps businesses identify specific needs, preferences, and purchasing behaviours, enabling them to create customised products or services that cater to their target market.
- This enhances customer satisfaction and loyalty.
Furthermore, data collection allows businesses to optimise their strategies.
- By continuously monitoring and analysing data, organisations can identify areas for improvement, spot potential bottlenecks, and make data-driven decisions to streamline operations and enhance efficiency.
- This leads to cost savings, improved resource allocation, and a competitive advantage.
In summary, data collection is essential for businesses to thrive in today’s customer-centric market.
- It enables businesses to gain valuable insights, tailor offerings, enhance customer experiences, and refine overall strategies.
- Embracing data collection practices helps businesses stay ahead of the competition and ensure long-term success with their marketing efforts.
1.3. Methods for data collection
There are numerous methods available for collecting data, offering flexibility and adaptability to different research needs. Commonly used methods include digital analytics, tracking tools, surveys, interviews, observation, and experiments. Each method offers unique advantages and should be chosen based on specific data requirements, target audience, and business objectives.
- Digital analytics and tracking tools have become significant in the era of technology. They allow businesses to collect and analyse data automatically, providing valuable information about user interaction, engagement, and preferences on websites, social media platforms, and mobile applications.
- Surveys involve gathering information through a set of questions administered to a sample of individuals. They can be conducted online, through mail, or in person, providing a wide reach and diverse responses. Interviews involve direct communication between the researcher and the participant, offering more detailed insights.
- Observation involves watching and recording behaviours, actions, and interactions in real time. It can be done in controlled environments or natural settings, providing valuable insights into human behaviour and societal patterns.
- Experiments are used to test hypotheses and understand cause-and-effect relationships. They involve manipulating variables under controlled conditions and measuring the resulting outcomes. Experiments provide insights into the impact of specific factors on desired outcomes.
By considering these various data collection methods, researchers can ensure comprehensive and diverse data collection, leading to a comprehensive understanding of the research topic and better-informed decision-making.
1.4. Tools for collecting data
Digital analytics and tracking tools like Web Analytics tools like Google Analytics, Piwik Pro and Snowplow, CRM platforms like HubSpot and Salesforce, and survey tools like SurveyMonkey have become indispensable in modern data collection efforts. These tools offer valuable insights and enable organisations to gather and analyse data more effectively.
Many marketers and website owners choose to use a Tag Management system to collect data from their websites, this enables data collection with a unified and structured approach. Many vendors provide their own Tag Management solutions, however, most of the solutions like Google Tag Manager, Piwik Pro Tag Manager and Tealium iQ Tag Manager are destination agnostic and can be used to send data to any required destination.
1.5. Things to consider when collecting data
To maintain ethical data collection practices and adhere to legal requirements, it is crucial to consider certain factors.
- Familiarise yourself with laws such as the General Data Protection Regulation (GDPR) to ensure compliance.
- Align your data collection efforts with your specific business goals.
- Collect data that is relevant to your objectives to gain valuable insights for the success of your organisation.
Keeping up with the latest developments in data privacy and protection is important.
- Stay informed about any changes or updates to data protection laws and regulations to ensure compliance.
- Regularly review and update your data collection processes and policies to align with best practices and industry standards.
Obtaining consent from individuals whose data you collect is essential.
- Have clear and transparent processes for obtaining consent and provide individuals with the option to opt-out or withdraw their consent at any time.
- Respect individuals’ privacy rights and handle their data with care and confidentiality.
Data security is critical.
- Implement robust security measures to protect collected data from unauthorised access, loss, or theft. This includes using encryption, secure storage systems, and access controls to safeguard sensitive information.
- Regularly conduct security audits and assessments to identify and address vulnerabilities in your data collection and storage processes.
Consider the ethical implications of your data collection practices.
- Be transparent about how you collect and use data, and use it responsibly and ethically.
- Avoid using data for purposes unrelated to your business goals or that could potentially harm individuals or violate their rights.
By considering these important factors, you can ensure that your data collection practices are ethical, legal, and aligned with your business goals, ultimately contributing to the success and reputation of your organisation.
Read our marketing ethics consumer report to learn more about how you can keep consumer experiences top of mind in your digital marketing strategy.
2. How to store data
2.1. What is data storage?
Data storage is the process of archiving collected data in various digital repositories, databases, or other storage solutions. It ensures the secure storage and easy accessibility of data when needed.
Effective data storage practices are crucial for organisations to efficiently manage and utilise their vast amounts of information. By implementing robust data storage systems, businesses can ensure the integrity, accessibility, and long-term preservation of their valuable data assets, enabling informed decisions and valuable insights for their operations and strategic planning.
2.2. Types of data storage
Businesses have a range of options to consider when deciding between on-premise solutions, cloud storage, or hybrid systems. Most marketing platforms and data solutions offer their data storage options, but it is often advisable to extract the data from the platforms and store it in a data warehouse or data lake, to make it possible to combine and analyse in one place.
Market leaders in the cloud space include Google Cloud Platform, Amazon Web Services, Snowflake and Microsoft Azure.
- The choice should be carefully evaluated based on factors such as the size of the business, specific needs, and available budget.
- Assess the scalability, security, and reliability of each option to ensure alignment with long-term goals.
- Consider factors like data accessibility, ease of implementation, and maintenance requirements to make an informed decision.
By taking these factors into account, businesses can select the most suitable solution that effectively meets their requirements and supports growth.
2.3. How does data storage work?
Data is stored in structured formats within databases or other systems, enabling convenient retrieval and efficient analysis. This is often achieved with the concept of Data Contracts. Data Contracts sets standards within an organisation for how data is structured, formatted and used for various purposes. This allows for seamless integration between applications and facilitates data sharing across platforms.
Structured data storage ensures data integrity and enhances data security measures, safeguarding sensitive information from unauthorised access. By utilising structured data storage and data contracts, organisations can streamline operations, optimise data management processes, and make data more readily available within the organisation.
2.4. Benefits of managed data storage
Effective storage solutions safeguard data and ensure seamless retrieval where required. A managed solution like Google Cloud, Redshift or Snowflake provides safeguards and redundancies while making it possible to interact with your data via secure connections in the contexts you need it, whether this is for reporting, machine learning models or data enrichment in other platforms.
These solutions also enable businesses to scale without encountering data management issues. Reliable storage systems protect valuable information, streamline operations, and support future growth.
2.5. Things to consider when storing marketing data
Ensuring the safety and security of data is paramount in today’s digital landscape.
- Implement robust and secure storage solutions to protect sensitive information from unauthorised access.
- Consider backing up your data regularly to ensure data resilience and a quick recovery in the event of incidents.
- Organisations must remain compliant with data storage regulations to avoid legal implications and maintain customer trust.
- Emphasise safety measures to safeguard valuable data assets and maintain the integrity and confidentiality of information.
3. How to activate data
3.1. What is a data activation platform?
A data activation platform is a sophisticated technology solution that transforms passive data into valuable insights. By leveraging this platform, businesses can unlock the full potential of their data, resulting in improved marketing outcomes and enhanced customer experiences. This platform serves as a powerful tool that enables organisations to analyse, interpret, and utilise data strategically, leading to informed decision-making and a competitive edge.
3.2. Stages of data activation in marketing
Data activation involves several stages in transforming raw data into actionable strategies:
- Data collection: Acquire raw data from various sources, including databases, surveys, and customer interactions.
- Data integration: Combine and organise the collected data into a unified dataset, eliminating duplicates and ensuring consistency.
- Data analysis: Analyse the integrated data to extract valuable insights and patterns using advanced analytical techniques.
- Strategy development: Develop strategies from data insights for effective business solutions.
- Strategy application: Implement the formulated strategies, such as executing marketing campaigns or making data-driven decisions across business functions.
By following these stages, organisations can leverage their data assets effectively to gain a competitive advantage and drive success in their marketing efforts.
3.3. Benefits of data activation in marketing
With data separated in silos divided by the platforms that produce the data, very little can be done to activate key pieces of data in your marketing efforts. This can result in disconnected marketing efforts that are reaching the wrong targets at the wrong time.
Data activation helps address these challenges faced by businesses. It helps create tailored marketing campaigns, significantly improves customer segmentation, and provides valuable insights and analytics for informed and strategic decision-making.
In other words, data activation in marketing plays a pivotal role in enhancing overall business performance and driving growth.
3.4. Steps to creating a comprehensive and effective data activation strategy
Creating a successful data activation strategy involves several key steps:
- Define clear and specific goals (KPIs).
- Integrate and centralise your data.
- Analyse and segment your data.
- Apply insights to marketing strategies.
- Measure and refine regularly
By following these steps, you can create a comprehensive and effective data activation strategy that helps achieve marketing objectives.
Conclusion & further reading
Mastering data collection, storage, and activation is essential for success in marketing. Aligning these processes with a shared goal is key, providing insights into consumer behaviour and market trends. Ethical and legal considerations are imperative, ensuring practices align with consent, privacy, and security. Effective storage, whether on-premise or in the cloud, is crucial for seamless data retrieval.
Data activation transforms raw data into actionable insights, contributing to strategic decision-making and business growth. Creating a comprehensive strategy involves clear goal definition, data integration, analysis, segmentation, and regular refinement. In today’s data-driven world, mastering these aspects provides a competitive edge.
For a deeper dive into each topic, explore our comprehensive guides linked within the respective sections. Grasping the art of data collection, storage, and activation will provide a competitive edge in today’s data-driven world.
What is that? You want further reading? You got it: