Dimensions and metrics in Google Analytics – moving beyond the standard setup
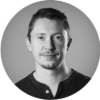
Christopher Beardsley
Analytics Specialist
Dimensions and Metrics form the basis of what Google Analytics data is built upon. Out of the box, Google Analytics offers many to choose from enabling you to answer a number of critical business questions related to your website. But, what if you need to answer questions that the built-in combinations of dimensions and metrics don’t cover?
The evolution of Google Analytics
Google Analytics has been around since 2005 when Google acquired the web analysis and statistics program Urchin before launching Google Analytics in November of that year. That’s a whole 14 years ago now which is a lifetime in such a rapidly changing industry. We’ve seen many updates to GA over the years and, to this day, Google Analytics continues to innovate and develop, helping ensure GA retains its position as the go-to analytics solution for the vast majority of site owners and marketers alike. Most recently, Google Analytics underwent possibly its biggest makeover since that launch back in November 2005, introducing the aptly named Google Analytics App & Web property, described in detail here by our UK CEO Rhys Cater. Despite the push towards GA App & Web property, GA standard web property remains, for the time being at least, the recommended primary solution for web analysis in the Google stack.
Out of the box, Google Analytics is a very useful analytics solution. It offers a vast array of useful reports and graphs. For many sites, a standard GA implementation (with little to no custom implementations) will help to answer a number of the business questions related to the website and will, to a certain degree, suffice. The downside to an out-of-the-box implementation is something Google looked to tackle as part of the introduction of Universal Analytics back in 2012, namely the ability to slice and dice your data with your own dimensions.
So, what is a dimension?
As per Google, “Dimensions are attributes of your data. For example, the dimension City indicates the city, for example, “Paris” or “New York”, from which a session originates. The dimension Page indicates the URL of a page that is viewed”.
Google Analytics OOTB (out-of-the-box!) offers a generous 100+ dimensions which can be found and explored here. Some of the dimensions available are, for example:
- Browser: Examples: Safari, Chrome
- Device category: Examples: Desktop, Mobile, Tablet
- User type: Examples: New, Returning
Ok, so what are metrics?
Again, to use Google’s own definition, “Metrics are quantitative measurements. The metric Sessions is the total number of sessions. The metric Pages/Session is the average number of pages viewed per session.” A general rule of thumb is: if it’s text, it’s a dimension. If it’s a number, it’s a metric. So, based on the below image, we’re able to read the metric Users split by the dimension Browser, essentially answering the question, “how many users did we have using Chrome during the chosen time period?”. Using this report as an example, other questions you may want to answer here could be:
- “Why do Chrome users view more pages than, for example, Safari users?”
- “On the Opera browser, why are almost 80% of my users leaving after only viewing 1 page?”
Note that Google Analytics (and web analytics in general) is great at telling you the what but less great at telling you the why. Ignoring for a moment the fact that some of the above numbers are too low to draw any real conclusions, questions like the ones above could lead to you deciding to do some browser compatibility testing to ensure the site is as functional and user-friendly regardless of browser.
So, what was that about our own dimensions?
Despite the many built-in dimensions, there will come a point when you want to know more about your visitors and their on-site behaviour than what is available OOTB. For example, sure you have a user-type dimension so you can compare new versus returning users and their respective behaviours and actions on my site, but that dimension relies solely on cookie persistence which, unless you’ve been hiding under a rock for the past year or so, you’ll know is less reliable than it’s ever been due to changes in browser technologies.
So, you may instead want a logged-in status dimension which returns a true or false value to truly be able to analyse your logged in users’ activity versus those who are not logged in (caveat: a false value could well mean they are a previous customer but just not yet logged in. This is where Scope comes in. More on that another time…) I won’t go into any great detail in terms of how to set these values up, but in short, it requires having a developer push those dynamic values into the dataLayer whereby they can be grabbed and sent to GA together with any subsequent pageviews, events or transactions.
Or let’s say you want to see how often you’re driving traffic to products which are not in-stock, you might want to set a custom dimension at the product level called stock status which returns in-stock or out-of-stock accordingly. This could be vital in helping you determine the value of the traffic you’re driving to your site.
If you have a content site, you might otherwise decide you want to be able to analyse your visitors’ levels of engagement (or lack thereof) based on which authors have written the articles they are reading. A simple author custom dimension set at the page level would allow me to do that.
Which Custom Dimensions can help take your Digital Marketing efforts to the next level?
Aside from the above generic examples, here are some of my most used Custom Dimensions and ones any business should consider implementing, in my opinion:
UserId – Assuming you’ve already understood the importance in implementing the userId feature in Google Analytics (if not, you really should if your site has a signed-in state). On top of implementing userId as a feature and all the benefits that come with that, having it as a Custom Dimension is crucial when it comes to diving deeper into your user behaviour such as when doing CLV analysis, such as some of the examples my colleague Hanna writes about here. Implementing userId as a feature doesn’t by default expose the userId in the GA interface either, so this allows for a bit more analysis on a true user level, even in a non-userId view.
CLV (Customer Lifetime Value) – As well as performing CLV analyses on data outside of GA, it can often be beneficial to push that data back into GA as a custom dimension and bucket users based on CLV ranges, which can in turn be used to create audiences for remarketing lists. That’s on top of the many behavioural reports you’ll now have the ability to create with CLV range as your primary dimension.
nTh Purchase – As with CLV as a custom dimension, getting the index of a purchase into GA and a custom dimension opens up a plethora of analysis opportunities. Bucketing user behaviour based on which index purchase a user completed last is a great way to be able to analyse, for example, which marketing activities drove engagement and/or conversions for a first time purchaser compared with someone completing, say, their 10th-15th purchase. Or you may want to, for example, look at to what extent (or if at all) the size of the first purchase impacts the number (or size) of subsequent transactions.
Above, I mentioned that metrics are usually a number and dimensions usually a word and then I’ve gone and flipped that on its head with my examples above. Let me briefly explain why the above are, for certain use cases, more effective as dimensions and thus break my self-imposed rule.
Metrics, by default, aggregate over time. So, having CLV, for example, as a metric would behave very differently to how I need it to. CLV can obviously change over time due to actions the user takes on the site. If I set one CLV value today (example: 2000 SEK) and an updated value next month (let’s say 2500 SEK), the user would fall under the correct dimension as a dimension is updated with a new value whereas a metric for CLV in this instance would return 4500 SEK, which is not correct and skews my analysis.
All in all, Google Analytics is the market leader for a reason and its dominance shows no sign of relenting. Using a customised setup, tailored for your own business needs, is when Google Analytics power is truly unlocked.